A Journey with AI: From Speech to Finance
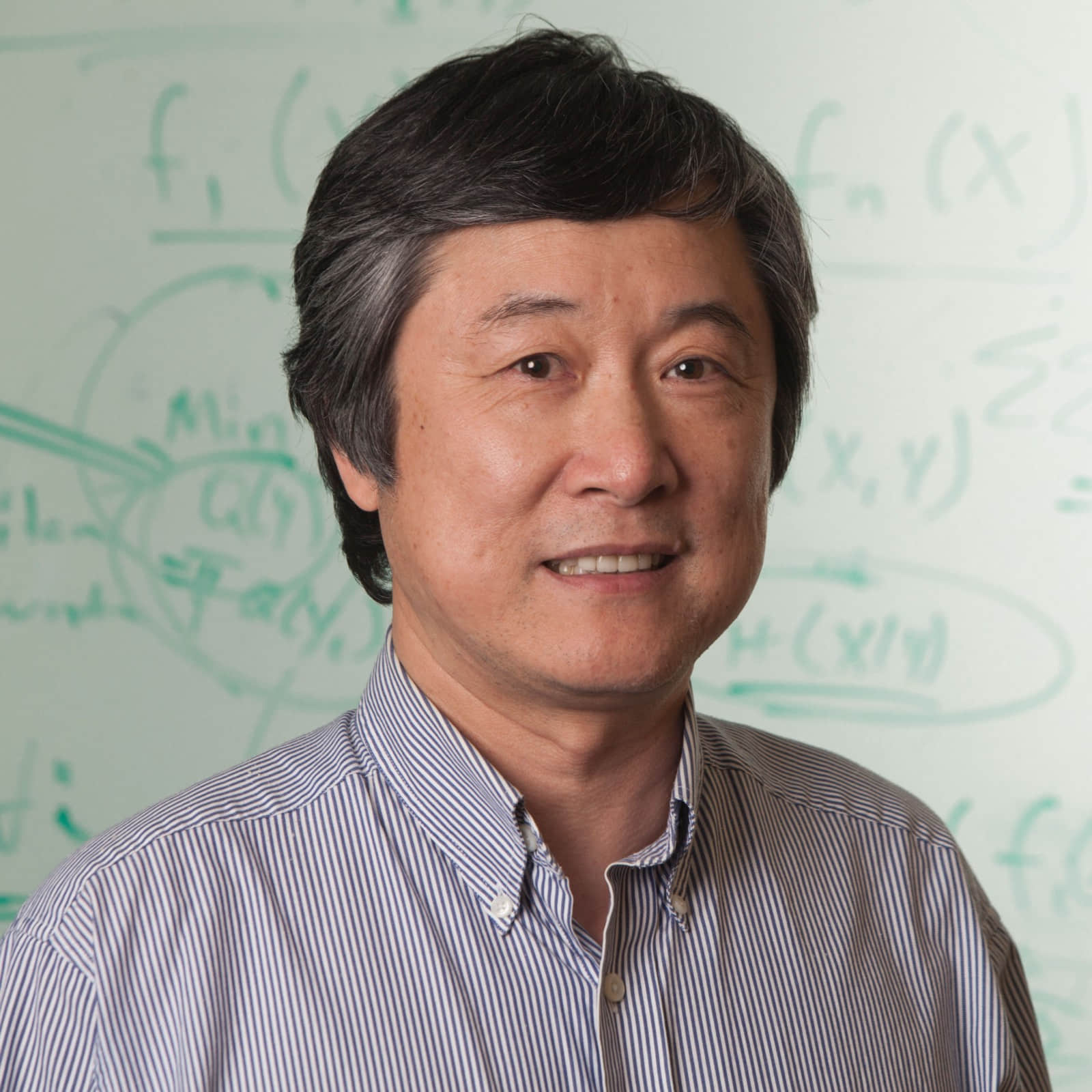
Li Deng’s journey with AI has spanned for more than 3 decades. After working as corporate researcher and university professor and built his career in speech research, Deng has taken a plunge into the financial world: He joined the $30 billion hedge fund Citadel as the Chief AI Officer in May 2017, leaving behind his job as a Chief Scientist of AI at Microsoft, where he headed the company’s AI school and founded the Deep Learning Technology Center. Also left behind was his affiliated professorship at the University of Washington for over 17 years.
Deng’s move was followed by other renowned AI professors. Manuela Veloso, Head of the Machine Learning Department at Carnegie Mellon University joined J.P. Morgan early this year, to lead the firm’s Artificial Intelligence Research Lab. Pedro Domingos, the University of Washington Computer Science Professor who authored the book The Master Algorithm, joined the DE Shaw Group hedge fund to lead its new Machine Learning Research Group just last week.
The financial world is seeing increasing adoption of AI since the vast amount of data available in the capital market. They call for sophisticated methods of machine learning to automate the data processing and prediction. “I feel that the finance industry is now pretty mature for AI to make an impact,” says Deng.
As an accomplished scientist, Deng has published more than 300 academic papers and authored several books on AI related topics. He is particularly vested in deep learning. Deng believes deep learning will soon be good at predicting the price of the future or making smart trading decisions.
The Early Years of Neural Networks
Li Deng attended the University of Wisconsin at Madison from 1982 to 1987 for his Ph.D. study in Electrical Engineering. During these years, he attempted to build neural models for human auditory simulation and speech recognition. Things did not go well because computers didn’t have enough computing power to effectively empower the work required by large neural networks, which became one of the reasons that stagnated Deng’s neural network research.
Deng did not give up. After joining University of Waterloo in Canada as an assistant professor (and stayed for the next 10 years), Deng and one of his students proposed a novel approach to enhance the memory of the neural network in 1993. While the model was a complete system, it still could not achieve better performance than the one with hidden Markov model.
The External Examiner of the doctoral thesis was Geoffrey Hinton, the undisputed pioneer of deep learning. He read the paper and told Deng that at this stage it was too difficult to achieve success in neural networks. The disappointing results pushed Deng away from the neural network research for many years. He turned to Bayesian statistical methods and generative models.
Applying Deep Neural Networks to Speech Recognition
Before the rise of deep learning, the shallow machine learning methods such as hidden Markov model (HMM) and Gaussian mixture model (GMM), have been dominating the field for almost 30 years.
Deng joined Microsoft in 2000 after leaving University of Waterloo and focused on speech recognition research using a Bayesian approach. But the result was not satisfying as the multilevel Bayesian networks exponentially increase computational complexity.
In 2006, Hinton published the paper “A fast learning algorithm for deep belief nets”. It suggested even with three hidden layers, the network still generates an well-behaved generative model of the joint distribution of handwritten digit images and their labels. Deng was fascinated by the results. He realized deep neural networks might have a shot in speech recognition.
At NIPS 2009, Deng and Dr. Hinton co-organized the workshop Deep Learning for Speech Recognition and Related Applications. For the first time, they proved that deep neural network trained with novel approaches can outperform previous methods on a variety of speech recognition benchmarks. The results are published in the paper “Deep Neural Networks for Acoustic Modeling in Speech Recognition” co-authored by Hinton and Deng in 2012.
“Deep learning is revolutionary in the sense that it actually disrupted the paradigm by allowing the representation of speech signal … in temporal domain and in the spatial domain,” Deng said.
In the following years, Deng and his team applied different deep learning techniques to speech, including LSTM recurrent neural networks, deep convolutional neural networks, sequence learning, and ensemble learning. These innovations dramatically boost speech recognition accuracy and are successfully integrated into speech understanding and speech translation.
In 2015, Deng was awarded the IEEE Signal Processing Society Technical Achievement Award for his outstanding contributions to the fields of deep learning and automatic speech recognition.
A New Adventure in Finance
Speech recognition technology has matured over the recent years. Deng is ready to take on the new challenge. He is now leading the AI efforts in Citadel, one of the world’s largest alternative asset managers with more than $30 billion in assets under management.
Citadel
Moving from speech recognition into finance was not easy though. While the two domains have some similarities — market data and speech data are both sequential in nature — financial data has some significant challenges:
- While the volume of market and capital data is growing exponentially, the amount of useful information indeed isn’t. The signal-to-noise ratio is much lower in finance which means most of the info is just noise. Then investors must separate the signal from the noise.
- Non-stationarity is another challenge. Because the financial market is a place where many participants compete against each other, there is a lot of fake data that have to be eliminated.
- The diversity of data is much bigger than speech data, such as text, images, speech, market data, and etc. “To solve the data problem, some of the economic and financial knowledge and model will have someplace to play,” says Deng.
Today, Deng’s research focuses on interpreting text data in machine translation system to improve quantitative investments. How to apply cutting-edge AI researches to current opportunities and challenges? We await to hear from Deng.
On Nov 10, 2018, Li Deng will speak at AI Frontiers Conference in San Jose, California. AI Frontiers Conference brings together AI thought leaders to showcase cutting-edge research and products. Besides Li Deng, other speakers include: Ilya Sutskever (Founder of OpenAI), Jay Yagnik (VP of Google AI), Kai-Fu Lee (CEO of Sinovation), Mario Munich (SVP of iRobot), Quoc Le(Google Brian), Pieter Abbeel (Professor of UC Berkeley) and more.
Get you ticket today. The early-bird price ends on August 31. For more information, please visit aifrontiers.com